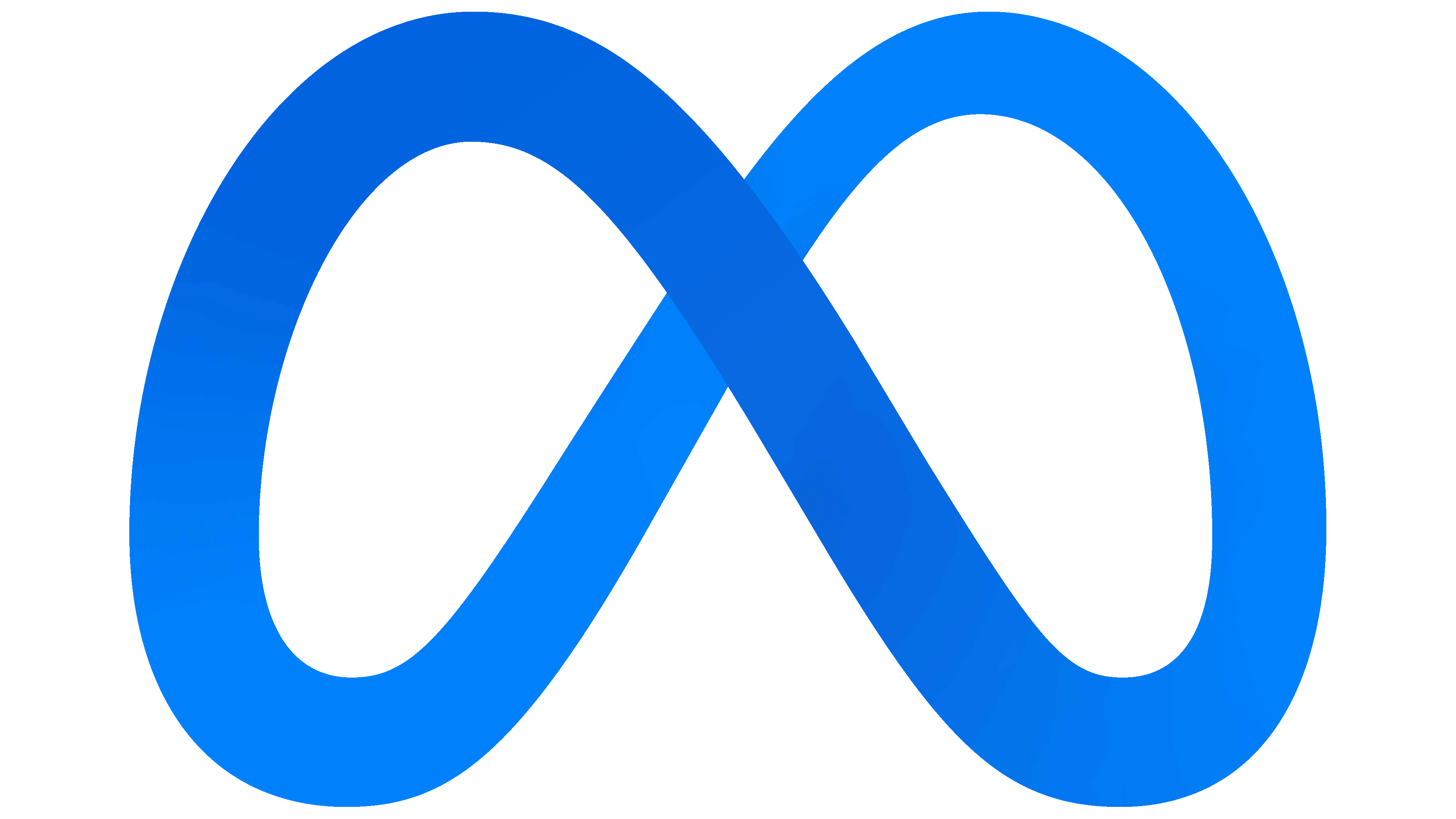

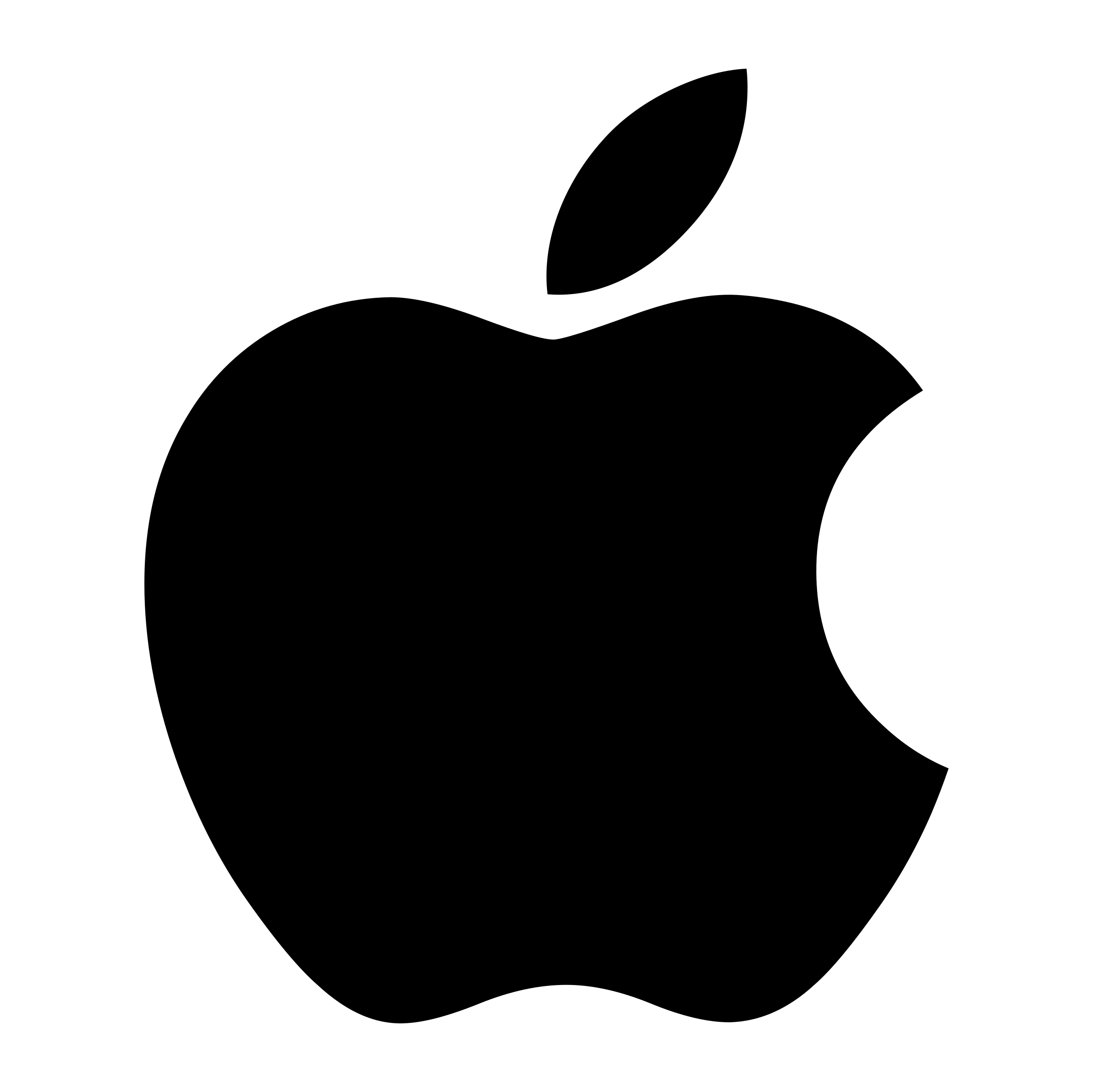
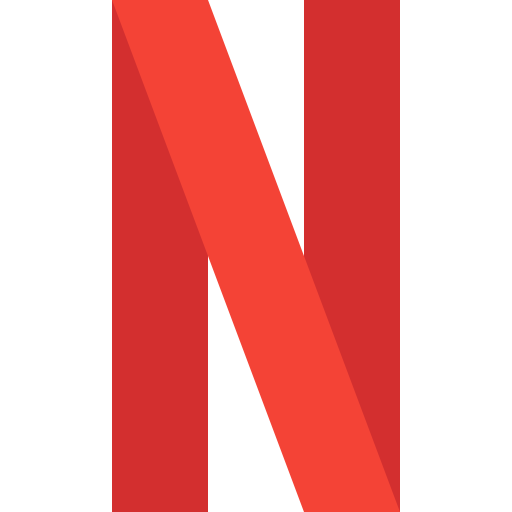
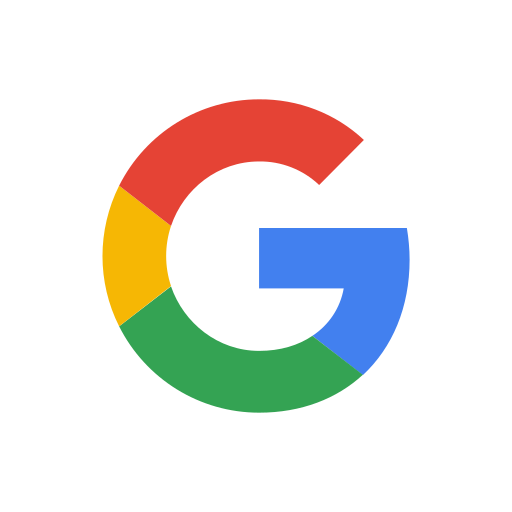
Jb Tak Fodega Nhi 🎯 Tb Tk Chodega
Nhi 📚 (MAANG)
DPL38 Best Time to Buy and Sell Stock IV
We are given an array Arr[] of length n. It represents the price of a stock on ‘n’
days. The following guidelines need to be followed:
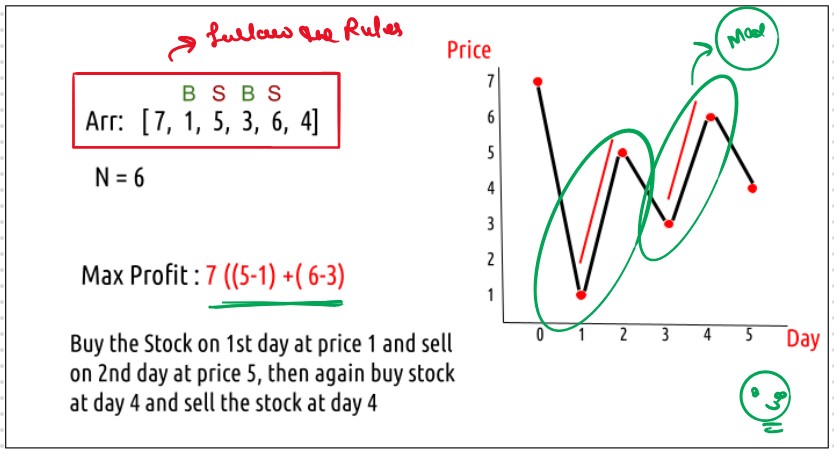
Find and return the maximum profit you can achieve.
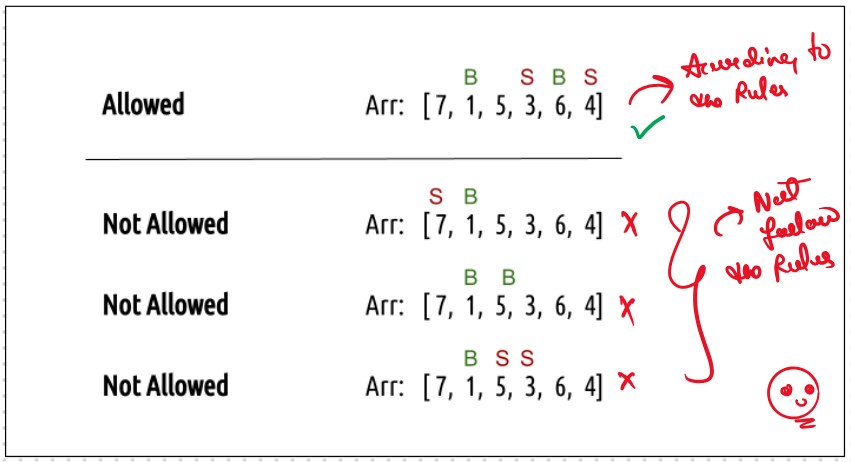
Only Transactions Updates in this Question As Compared to the Last, DPL37 Best Time to Buy and Sell Stock III
In Best Time to Buy and Sell Stock IV We Allow K Transactions In Best Time to Buy and Sell Stock III our Task is We Have Only 2 Transactions Allow, and this Problem We Allow the K Transactions this is the Only Change in This Proble and Entire Approch and Solution will Be Same As we Learned in Best Time to Buy and Sell Stock III
This Problem is the Application Varient of the Best Time to Buy and Sell Stock III,
Just Only Modified or You can Say Change the Value of the Transactions = 2 to Transactions = k
Remember Only Change is Transactions = 2 to Transactions = K as we Learned in the Best Time to Buy and Sell Stock III
Now Remaining Entire 👇 Process will Be Same As Like in DPL37

In Recursice Approch We Just Add the Cap Variable in the Recursion,
Memoization, tabluation and Space Optimization that Show the Limit of the 2, and
Modified Entire Solution on the Base of the Cap Variable
Now Here is the Question Why we:-
the Answe is Any transaction Whern Counted Completed, When we Buy and Sell the Stock And Gain Some Profit, So we Need to Change the Cap Value, and When we Not Buy and Sell the Stock, So we Not Need to Change the Cap Value. Buy Stock + Shell Stock = Count 1 Transaction
Now Remaining Entire 👇 Process will Be Same As Like in DPL36
Every day, we will have two choices, either to do nothing and move to the next day or to buy/sell (based on the last transaction) and find out the profit. Therefore we need to generate all the choices in order to compare the profit. As we need to try out all the possible choices, we will use recursion.
Recursice Approch
Again here we follow the out Standerad Patter that we learned in the Dynamic Programming Introduction. How to Write Recursion
Steps to form the Recursive SolutionStep 1: Express the problem in terms of indexes.
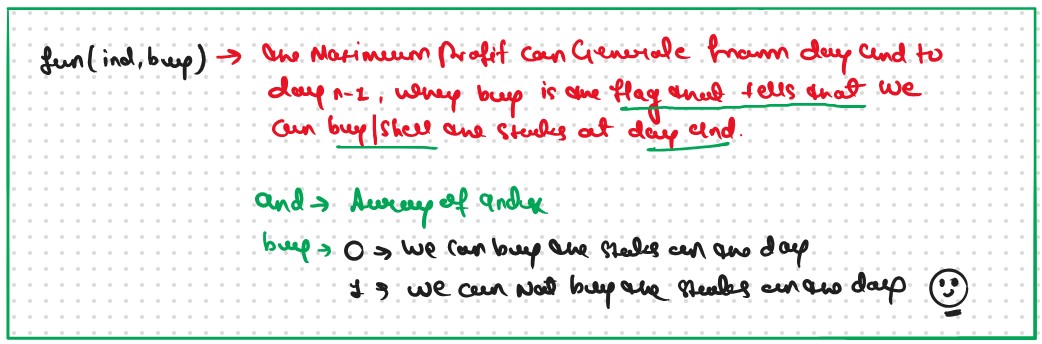
Step 2: Try out all possible choices at a given index.
Every day, we have two choices
If we can buy the stock on a particular day, we have two options
Case 2: When buy == 1, we can sell the stock.
Agin we have If we can buy the stock on a particular day, we have two options
The figure below gives us the Summary
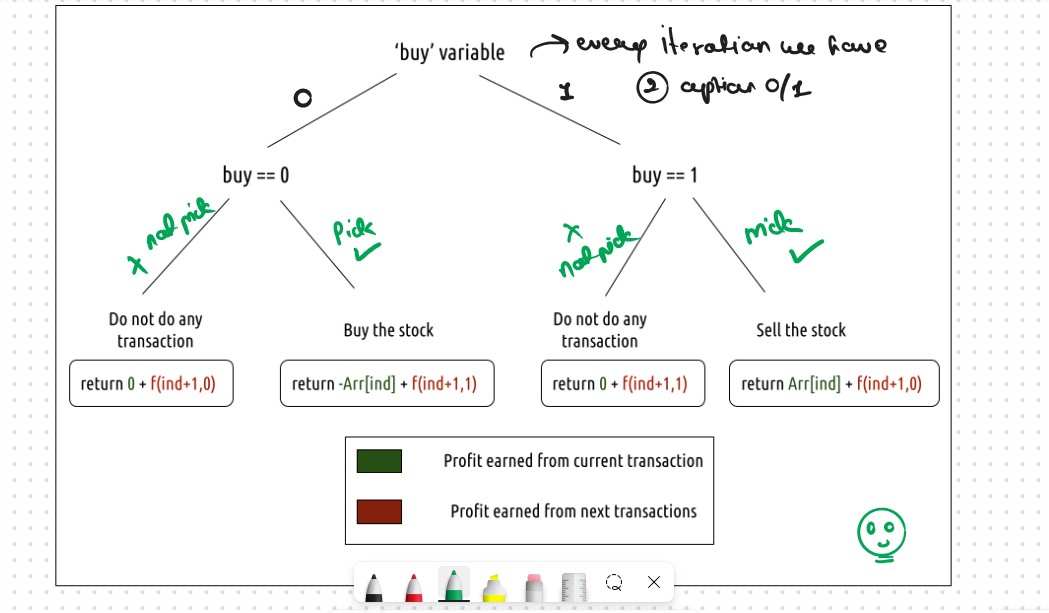
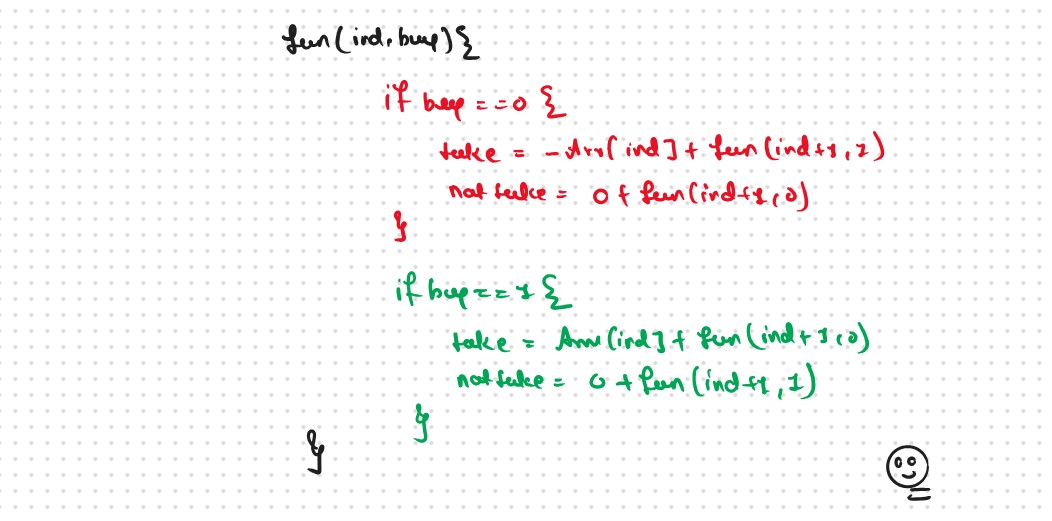
Step 3: Return the Maximum
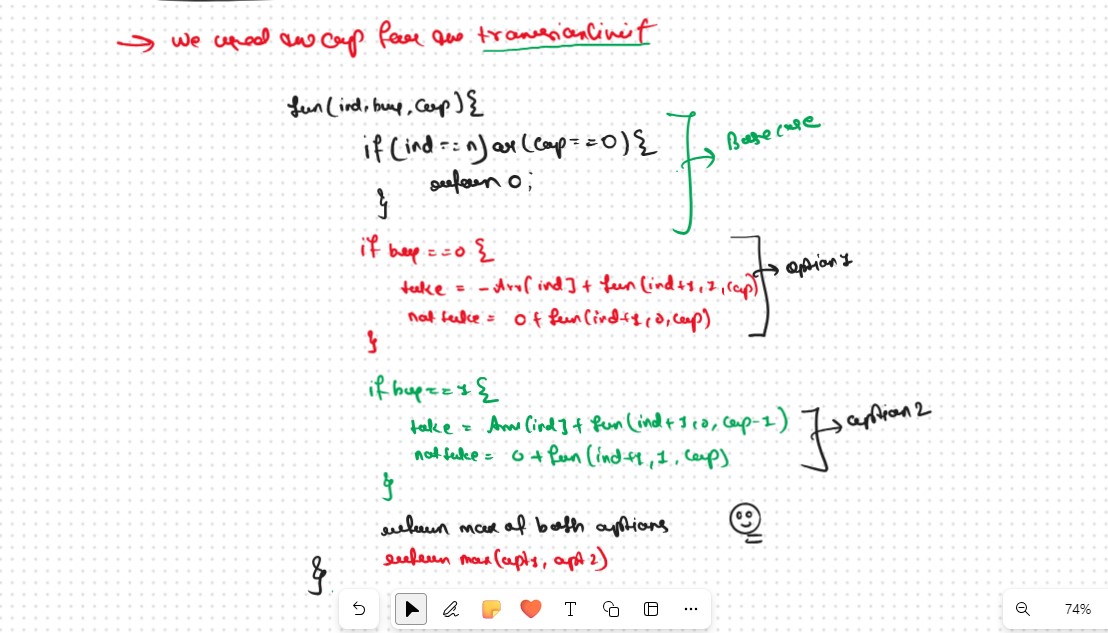
Base Cases
class Solution: def RecursionApproch(self, prices,index,n,decisionFlag,transactionLimit): # Base Case if index == n or transactionLimit == 0: return 0 maxProfit = 0 if decisionFlag == 1: # as similar to take and notTake Concepts buyTheStock = -prices[index] + self.RecursionApproch(prices,index+1,n,0,transactionLimit) notBuyTheStock = 0 + self.RecursionApproch(prices,index+1,n,1,transactionLimit) maxProfit = max(buyTheStock,notBuyTheStock) else: shellTheStock = prices[index] + self.RecursionApproch(prices, index + 1, n, 1,transactionLimit-1) notSellTheStock = 0 + self.RecursionApproch(prices, index + 1, n, 0,transactionLimit) maxProfit = max(shellTheStock, notSellTheStock) return maxProfit def maxProfit(self, prices,K): n = len(prices) decisionFlag = 1 transactionLimit = K return self.RecursionApproch(prices,0,n,decisionFlag,transactionLimit) ans = Solution() prices = [2,4,1] K = 2 print(ans.maxProfit(prices,K))
Time & Space Complexity
Time Complexity: O(2^N)Reason: Exponential Time we find out the all the Possible Path
Space Complexity: O(N+K)
Reason: We are using a recursion stack space(O(N+K))
Memoization Approch
If we Observe in the recursion tree, we will observe a many number of overlapping subproblems. Therefore the recursive solution can be memoized for to reduce the time complexity.
Steps to convert Recursive code to memoization solution:Where:-
n:- Size of the Input Array
2:- Dession Flag
3:- Cap Flag or Transaction Limit
class Solution: def MemoizationApproch(self, prices,index,n,decisionFlag,dp,transactionLimit): # Base Case if index == n or transactionLimit == 0: return 0 if dp[index][decisionFlag][transactionLimit] != -1: return dp[index][decisionFlag][transactionLimit] maxProfit = 0 if decisionFlag == 1: # as similar to take and notTake Concepts buyTheStock = -prices[index] + self.MemoizationApproch(prices,index+1,n,0,dp,transactionLimit) notBuyTheStock = 0 + self.MemoizationApproch(prices,index+1,n,1,dp,transactionLimit) maxProfit = max(buyTheStock,notBuyTheStock) else: shellTheStock = prices[index] + self.MemoizationApproch(prices, index + 1, n, 1,dp,transactionLimit-1) notSellTheStock = 0 + self.MemoizationApproch(prices, index + 1, n, 0,dp,transactionLimit) maxProfit = max(shellTheStock, notSellTheStock) dp[index][decisionFlag][transactionLimit] = maxProfit # we can also return maxProfit its also give the correct answer return dp[index][decisionFlag][transactionLimit] def maxProfit(self, prices,K): n = len(prices) decisionFlag = 1 transactionLimit = K dp = [[[-1 for _ in range(transactionLimit+1)] for _ in range(2)] for _ in range(n)] return self.MemoizationApproch(prices,0,n,decisionFlag,dp,transactionLimit) ans = Solution() prices = [3,2,6,5,0,3] K = 2 print(ans.maxProfit(prices,K))
Time & Space Complexity
Time Complexity:O(N*2*3)Reason: There are N*2*3 states therefore at max ‘N*2*3’ new problems will be solved
Space Complexity: O(N*2*K) + O(N)
Reason: We are using a recursion stack space(O(N)) and a 3D array ( O(N*2*K))..
Tabulation Approch
Tabulation is a ‘bottom-up’ approach where we start from the base case and reach the final answer that we want and Memoization is the Top-down Approch.In Tabulation Approch We Just Creat a DP Array Same as Memoization and Simply Convert the Recurance Relation into the form of the Looping
VVVV Important Point: Tabulation Approch is Always 1-Based Indexing Always RememberSteps to convert Recursive Solution to Tabulation one.
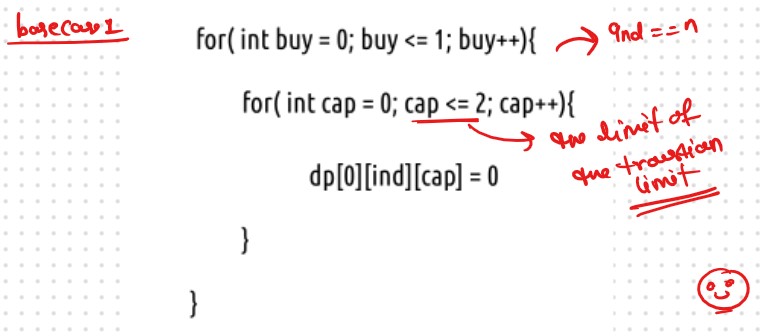
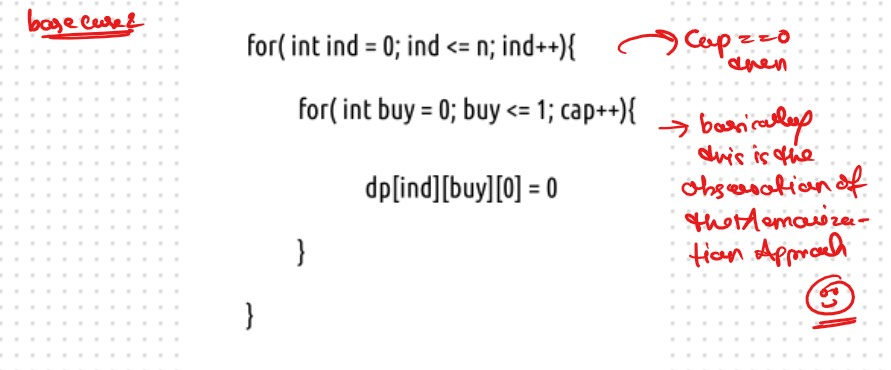
VVVVVVV Imp Point
class Solution: def TabulationApproch(self, prices,n,dp,K): # Base Case # if index == n for index in range(2): for currdecisionFlag in range(K+1): dp[0][index][currdecisionFlag] = 0 # if transactionLimit == 0 for index in range(n): for currdecisionFlag in range(2): dp[index][currdecisionFlag][0] = 0 print(dp) # Important Note: We All Ready in our DP inilisezed with the 0, no need to write the Base # Case allready all 0, But we Write the Base Case for the Understanding the Tabulation for index in range(n-1,-1,-1): for decisionFlag in range(2): for transactionLimit in range(1,K+1): maxProfit = 0 if decisionFlag == 1: # as similar to take and notTake Concepts buyTheStock = -prices[index] + dp[index + 1][0][transactionLimit] notBuyTheStock = 0 + dp[index + 1][1][transactionLimit] maxProfit = max(buyTheStock,notBuyTheStock) else: shellTheStock = prices[index] + dp[index + 1][1][transactionLimit-1] notSellTheStock = 0 + dp[index + 1][0][transactionLimit] maxProfit = max(shellTheStock, notSellTheStock) dp[index][decisionFlag][transactionLimit] = maxProfit # we can also return maxProfit its also give the correct answer print(dp) return dp[0][1][K] def maxProfit(self, prices,K): n = len(prices) transactionLimit = K dp = [[[0 for _ in range(transactionLimit+1)] for _ in range(2)] for _ in range(n+1)] print(dp) return self.TabulationApproch(prices,n,dp,K) ans = Solution() prices = [3,2,6,5,0,3] K = 2 print(ans.maxProfit(prices,K))
Time & Space Complexity
Time Complexity: O(N*2*K)Reason: There are three nested loops that account for O(N*2*K) complexity.
Space Complexity: O(N*2*K)
Reason:We are using an external array of size ‘N*2*K’. Stack Space is eliminated.
Space Optimization
If we closelly Observed if any Tabulation Approch we used the Some Limited Stuff like: dp[ind][buy][cap] = max(dp[ind+1][buy][cap] , max( dp[ind+1][!buy][cap])) for the finding the our ans then definetly here Spaced Optimization is Possible in that types of Problems. Always Remember
Golden Rule
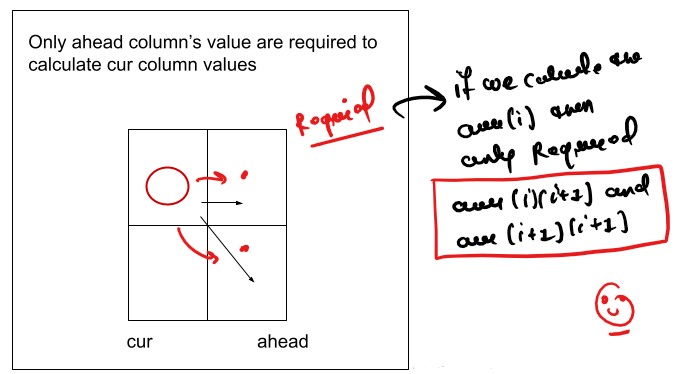
class Solution: def SpaceOptimizationApproch(self, prices,n,prev,curr,K): # Base Case # if index == n for index in range(2): for currdecisionFlag in range(K+1): prev[0][currdecisionFlag] = 0 # if transactionLimit == 0 for index in range(n): for currdecisionFlag in range(2): prev[currdecisionFlag][0] = 0 print(prev) print(curr) # Important Note: We All Ready in our DP inilisezed with the 0, no need to write the Base # Case allready all 0, But we Write the Base Case for the Understanding the Tabulation for indx in range(n-1,-1,-1): for decisionFlag in range(2): for transactionLimit in range(1,K+1): maxProfit = 0 if decisionFlag == 1: # as similar to take and notTake Concepts buyTheStock = -prices[indx] + prev[0][transactionLimit] notBuyTheStock = 0 + prev[1][transactionLimit] maxProfit = max(buyTheStock,notBuyTheStock) else: shellTheStock = prices[indx] + prev[1][transactionLimit-1] notSellTheStock = 0 + prev[0][transactionLimit] maxProfit = max(shellTheStock, notSellTheStock) curr[decisionFlag][transactionLimit] = maxProfit prev = curr # we can also return maxProfit its also give the correct answer return prev[1][K] def maxProfit(self, prices,K): n = len(prices) prev = [[0 for _ in range(3)] for _ in range(2)] curr = [[0 for _ in range(3)] for _ in range(2)] return self.SpaceOptimizationApproch(prices,n,prev,curr,K) ans = Solution() prices = [2,4,1] K = 2 print(ans.maxProfit(prices,K))
Time & Space Complexity
Time Complexity: O(N*2*K)Reason: There are three nested loops that account for O(N*2*K) complexity
Space Complexity: O(K)
Reason: We are using two external arrays of size ‘2*K’.
Most Space Optimization (That we Learned in DP on Stock III)
Now as we Discuss in the Optimial Approch in the DP on Stock III that is
If we observe very carefully, then its Possible to Optimized our Space frpm 3D DP to (N*4) DP Memoization, Tabulation and Space Optimized Possible ley's Understand How ?
What is N * 4 DP Solution
In our Solution what we do fun(cap,transcationLimit) if transcationLimit == 0
then we formed our Solution that we Discuss
So This Approch is the Completely Observation Based Approch
We know that we have the 2 transcationLimit that means 4 Options
Buy Shell Buy Shell 0 1 2 3
So here we Observed very Carefully our buy transcation will only then index % 2 == 0 (even) otherwise shell
That is the Core Concept that we used to Convert the our 3D DP Solution into the 2D DP Solution in All the Formate Memoization, Tabulation and Space Optimized
If I try to Integrating this Concept in this Solution then we See Very Carefully
VVVVVV Imp Point
Becouse the Base Condition is n to 0 and transcation == 0
Becouse the Base Condition is 0 to n and transcation == K
class Solution: def RecursionApproch(self, prices,index,n,transactionLimit,k): # Base Case if index == n or transactionLimit == 2*k: return 0 maxProfit = 0 if transactionLimit % 2 == 0: # as similar to take and notTake Concepts buyTheStock = -prices[index] + self.RecursionApproch(prices,index+1,n,transactionLimit+1,k) notBuyTheStock = 0 + self.RecursionApproch(prices,index+1,n,transactionLimit,k) maxProfit = max(buyTheStock,notBuyTheStock) else: shellTheStock = prices[index] + self.RecursionApproch(prices, index + 1, n ,transactionLimit+1,k) notSellTheStock = 0 + self.RecursionApproch(prices, index + 1, n ,transactionLimit,k) maxProfit = max(shellTheStock, notSellTheStock) return maxProfit def maxProfit(self, prices,k): n = len(prices) transactionLimit = 0 return self.RecursionApproch(prices,0,n,transactionLimit,k) ans = Solution() prices = [3,2,6,5,0,3] k = 2 print(ans.maxProfit(prices,k))
class Solution: def MemoizationApproch(self, prices, index, n, dp, transactionLimit,k): # Base Case if index == n or transactionLimit == 2*k: return 0 if dp[index][transactionLimit] != -1: return dp[index][transactionLimit] maxProfit = 0 if transactionLimit % 2 == 0: # as similar to take and notTake Concepts buyTheStock = -prices[index] + self.MemoizationApproch(prices,index+1,n,dp,transactionLimit+1,k) notBuyTheStock = 0 + self.MemoizationApproch(prices,index+1,n,dp,transactionLimit,k) maxProfit = max(buyTheStock,notBuyTheStock) else: shellTheStock = prices[index] + self.MemoizationApproch(prices, index + 1, n ,dp,transactionLimit+1,k) notSellTheStock = 0 + self.MemoizationApproch(prices, index + 1, n ,dp,transactionLimit,k) maxProfit = max(shellTheStock, notSellTheStock) dp[index][transactionLimit] = maxProfit # we can also return maxProfit its also give the correct answer return dp[index][transactionLimit] def maxProfit(self, prices,k): n = len(prices) transactionLimit = 0 dp = [[-1 for _ in range(2*k)] for _ in range(n)] return self.MemoizationApproch(prices, 0, n, dp, transactionLimit,k) ans = Solution() prices = [3,2,6,5,0,3] k = 2 print(ans.maxProfit(prices,k))
class Solution: def TabulationApproch(self, prices,n,dp,k): # Base Case # if index == n for index in range(2*k+1): dp[n][index] = 0 # if transactionLimit == 0 for index in range(n+1): dp[index][2*k]= 0 print(dp) # Important Note: We All Ready in our DP inilisezed with the 0, no need to write the Base # Case allready all 0, But we Write the Base Case for the Understanding the Tabulation for index in range(n-1,-1,-1): for transactionLimit in range(2*k-1,-1,-1): maxProfit = 0 if transactionLimit % 2 == 0: # as similar to take and notTake Concepts buyTheStock = -prices[index] + dp[index + 1][transactionLimit+1] notBuyTheStock = 0 + dp[index + 1][transactionLimit] maxProfit = max(buyTheStock,notBuyTheStock) else: shellTheStock = prices[index] + dp[index + 1][transactionLimit+1] notSellTheStock = 0 + dp[index + 1][transactionLimit] maxProfit = max(shellTheStock, notSellTheStock) dp[index][transactionLimit] = maxProfit # we can also return maxProfit its also give the correct answer print(dp) return dp[0][0] def maxProfit(self, prices,k): n = len(prices) transactionLimit = 0 dp = [[0 for _ in range(2*k+1)] for _ in range(n+1)] print(dp) return self.TabulationApproch(prices,n,dp,k) ans = Solution() prices = [3,2,6,5,0,3] k = 2 print(ans.maxProfit(prices,k))
class Solution: def SpaceOptimizationApproch(self, prices,n,prev,curr,k): # Base Case # if index == n for index in range(2*k+1): prev[index] = 0 # if transactionLimit == 0 curr[2*k] = 0 print(prev) print(curr) # Important Note: We All Ready in our DP inilisezed with the 0, no need to write the Base # Case allready all 0, But we Write the Base Case for the Understanding the Tabulation for indx in range(n-1,-1,-1): for transactionLimit in range(2*k-1,-1,-1): maxProfit = 0 if transactionLimit % 2 == 0: # as similar to take and notTake Concepts buyTheStock = -prices[indx] + prev[transactionLimit+1] notBuyTheStock = 0 + prev[transactionLimit] maxProfit = max(buyTheStock,notBuyTheStock) else: shellTheStock = prices[indx] + prev[transactionLimit+1] notSellTheStock = 0 + prev[transactionLimit] maxProfit = max(shellTheStock, notSellTheStock) curr[transactionLimit] = maxProfit prev = curr # we can also return maxProfit its also give the correct answer return prev[0] def maxProfit(self, prices,k): n = len(prices) transactionLimit = 0 prev = [0]*(2*k+1) curr = [0]*(2*k+1) return self.SpaceOptimizationApproch(prices,n,prev,curr,k) ans = Solution() prices = [3,2,6,5,0,3] k = 2 print(ans.maxProfit(prices,k))
Time & Space Complexity
Time Complexity: O(N*2)Reason: There are two nested loops that account for O(N*2) complexity
Space Complexity: O(5)
Reason: We are using just Size of 5(len) DP 1D Array prev and curr.